How to scale image annotation cost-effectively
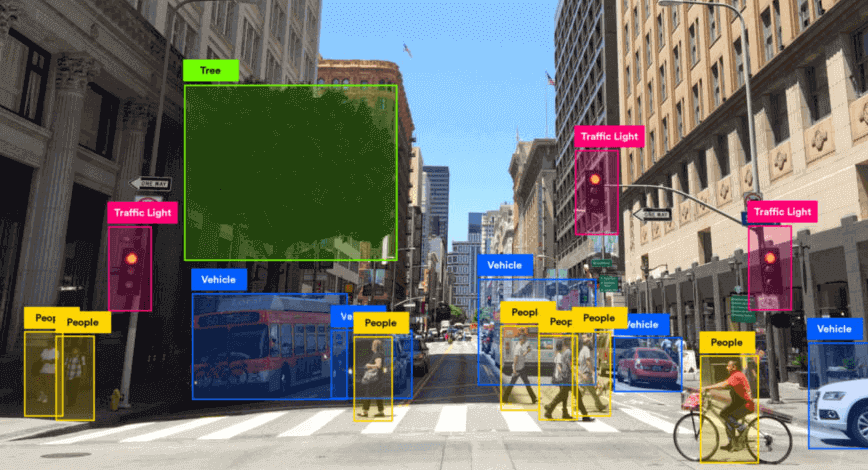
Visual data serves as the backbone of many advancements and innovations. In this regard, several AI technologies heavily rely on image annotation. For instance, accurately labeled image datasets are essential for powering computer vision systems, such as those used in autonomous vehicles. Image annotation involves meticulously labeling the visual content in each image using text for computer vision tasks.
However, the challenge lies in the fact that annotating images can be highly time-consuming and costly, particularly with large datasets.
Let’s delve into key considerations for efficiently scaling the image annotation process while maintaining budgetary constraints.
Best practices to scale image annotation cost-effectively
1. Leverage automated image annotation tools
While human annotation is essential for high-quality image labeling, integrating automated tools into the labeling process can significantly reduce costs and accelerate the procedure. Automated tools can help expedite the process by creating labels that can then be assessed by humans to verify their accuracy. This hybrid approach combines the efficiency of automation with human quality control. With this, businesses can streamline operations, minimize manual labor costs, and expedite the annotation workflow. Furthermore, automated labeling tools enable rapid processing of large image sets, primarily through advanced algorithms and machine learning techniques, facilitating the scaling of annotation workflows with minimal resource expenditure.
For instance, an eCommerce company selling furniture can use automated object detection models to rapidly identify and label common objects like chairs, tables, and lamps in product images. This automation reduces the need for manual annotation, saving costs. Human annotators can then focus on verifying and refining the annotated datasets, ensuring accuracy while optimizing resource allocation.
2. Crowdsource image annotation tasks
Crowdsourcing platforms like Amazon Mechanical Turk or Figure Eight offer access to a global pool of annotators, facilitating scalable and cost-effective image labeling solutions. By breaking down annotation tasks into smaller microtasks, businesses can harness the collective effort of numerous annotators, reducing both workload and costs for in-house teams. Moreover, crowdsourcing platforms typically feature competitive pricing structures, granting businesses flexibility in managing annotation expenses.
For instance, a self-driving car company can crowdsource the annotation of road elements, dividing tasks such as identifying vehicles, pedestrians, and traffic signs into microtasks. This approach accelerates the annotation process while minimizing the need for a large, dedicated in-house annotation team. By tapping into the diverse expertise of annotators worldwide, the company can obtain large volumes of annotated images. These labeled datasets will serve as training data to aid the company in various vehicle development aspects.
Also Read: Driving Efficiency Through B2B ECommerce: How to Build Sites That Enhance Business Operations
3. Optimize image annotation workflows
Optimizing image annotation workflows offers the advantage of increased annotator productivity and efficiency. By providing clear guidelines and user-friendly tools while streamlining processes, businesses can reduce the time and effort required for each image labeling task. This optimization leads to scaling annotation workflows cost-effectively. It enables annotators to work seamlessly, with minimal friction or distractions, and have access to resources that facilitate accurate and consistent labeling.
For instance, a retail company annotating product images can invest in user-friendly labeling tools with features like easy bounding box creation, polygon annotation, and attribute tagging, which can significantly improve annotator productivity. Clear guidelines and hotkey shortcuts can further streamline the annotation process, reducing the time and effort required for each task and ultimately lowering annotation costs.
4. Use a subset of images for annotations
Another practice to scale image annotation cost-effectively is by selecting a representative subset of a large image dataset that showcases the diversity, relevance, and key characteristics of the entire dataset for annotation. This approach, part of effective image annotation strategies, can significantly reduce annotation costs as businesses do not have to label an entire dataset. By prioritizing and labeling only the most relevant or informative images, businesses can optimize their annotation efforts and resources, thereby scaling their image annotation process efficiently.
For instance, an eCommerce company aiming to improve product image search and recommendations can strategically sample a diverse range of product images for annotation, out of a bulky image dataset. This sampling would include various categories, such as clothing, electronics, and home goods, as well as different angles, lighting conditions, and backgrounds, ensuring that the annotated sample captures the breadth of potential variations. By focusing annotation efforts on this representative subset, the company can train effective image recognition models while minimizing the need for exhaustive annotation of a large image dataset.
5. Leverage pre-trained models and transfer learning techniques
This image annotation practice can be specifically effective when working with limited annotation budgets as it reduces the amount of annotated data needed for training new models. By capitalizing on existing models trained on similar images, businesses can kickstart their annotation workflows with pre-labeled examples, effectively reducing the necessity for extensive manual labeling. These existing models have learned to recognize common patterns and features present in the images, providing a solid foundation for initializing annotation tasks. By fine-tuning these models for their target images, businesses can tailor the annotation process to better suit their unique needs and preferences.
For instance, an AI-powered educational platform can leverage pre-trained image classification models trained on educational datasets. These datasets may include images of textbooks, classroom settings, and educational materials, which significantly expedite the annotation process. This accelerates the development of their AI-powered platform and ensures that annotated images effectively cater to the educational context, providing better content recommendations and enhancing student engagement. Consequently, improving the overall learning experience for students.
6. Track and improve image annotation metrics
Close monitoring of various aspects of the annotation process ensures its efficiency and effectiveness are intact. Assessing the performance of annotation workflows, identifying areas for optimization, finding areas for automation, and implementing strategies to enhance overall productivity helps manage costs. Key metrics that businesses commonly track include annotation accuracy, consistency, turnaround time, and resource utilization. This analysis helps businesses take corrective actions to ensure reliability and coherence. They also get to focus on implementing measures to streamline workflows, ultimately aiding in cost-effective image labeling.
For instance, a healthcare firm developing AI-powered diagnostic solutions can track different metrics to gain insights into the efficiency of their image annotation workflows. In case the accuracy of annotation metrics dips below the desired threshold, they can swiftly intervene by providing additional training data or refining annotation guidelines, to improve the performance of the annotation models or algorithms. Similarly, if the turnaround time exceeds expectations, adjustments can be made to streamline processes or allocate more resources.
7. Outsource image annotation tasks
Delegating annotation responsibilities to external service providers or specialized annotation companies can offer several benefits, including cost savings, scalability, and access to expert annotators. Businesses can outsource image annotation tasks to reduce the burden on in-house teams, allowing them to focus on core business activities. Additionally, outsourcing provides access to a larger pool of skilled annotators, ensuring high-quality image labeling and faster turnaround times. It also allows businesses to scale annotation efforts according to fluctuating demand without the need for significant investments in infrastructure or hiring additional personnel. This flexibility enables businesses to adapt to changing project requirements and timelines effectively.
For instance, an automobile company developing advanced driver assistance systems (ADAS) and self-driving capabilities can outsource image annotation of road scenes to a service provider. The skilled team will accurately annotate diverse road environments, including labeling vehicles, pedestrians, traffic signs, lane markings, and other critical elements required for training ADAS and autonomous driving models. By outsourcing these intricate and labor-intensive annotation tasks, the automobile company can leverage the expertise and scalability of the service provider. As they ensure the delivery of high-quality image labeling services, the automobile company can free up internal resources to focus on core engineering and product development efforts.
In conclusion
Cost-effective image labeling is not just about reducing expenses but also about maximizing the value derived from annotated image datasets. By adopting these best practices, businesses can enhance the performance of their models and applications through high-quality annotated data, scale image labeling requirements, drive innovation, and gain a competitive edge in their respective industries.